Lucy Block, ANHD, will speak about shifting public narratives with data visualization
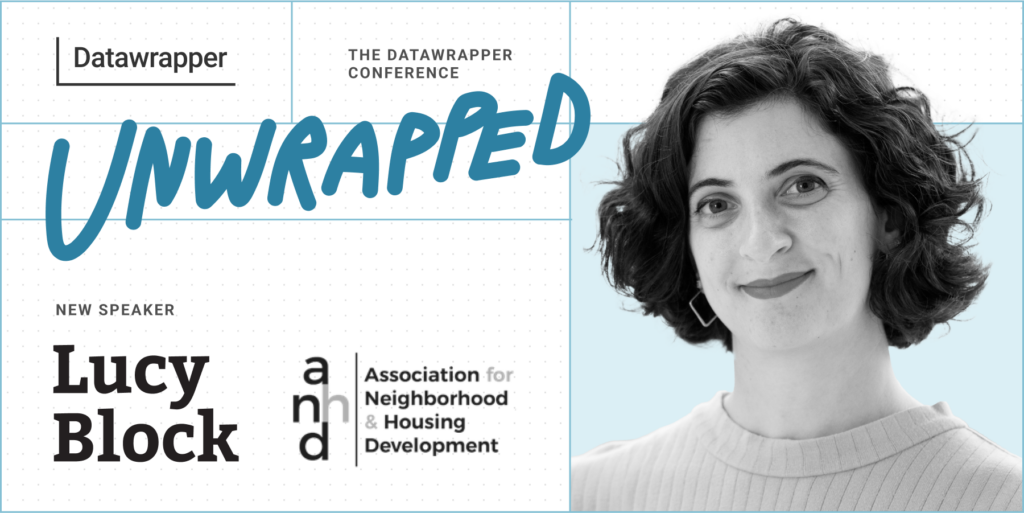
We’re excited to announce that Lucy Block from the Association for Neighborhood and Housing Development (ANHD) will speak at our Unwrapped conference about “Charting Change: How can data visualization help us win progressive policies?“
Lucy has been developing ANHD’s data analysis and storytelling work for six years. She uses many tools, but her favorite is Datawrapper. In addition, she manages ANHD’s Displacement Alert Project that includes the DAP Portal data tool. She also teaches NYU urban planning students how Datawrapper can help them tell powerful stories with data.
Time to ask her some questions:
Lucy, what will you talk about?
How can charts help you win progressive policies for social justice? My talk will demonstrate one New York City nonprofit organization’s approach to using Datawrapper to tell data stories that shift public narratives, convince elected officials, and fuel organizing campaigns with the goal of winning affordable housing and thriving, equitable neighborhoods for all New Yorkers.
The Association for Neighborhood and Housing Development, or ANHD, has a unique approach to incorporating charts and maps into our campaign messaging and policy work. We are not shy about having a point of view and policy objectives, and we use data analysis and visualization as a key tool in our storytelling to convey what New Yorkers need.
I'll walk you through my organization's internal uses of Datawrapper to quickly gain insights and iterate and our external uses to move local campaigns and policies.
How do you use Datawrapper?
One important way I use Datawrapper is to answer incoming data questions quickly and to give my teammates a sense of whether there's something worth pursuing. They might ask: How have New Yorker's incomes changed over the last few decades? How does that vary by race? I'll pull together the numbers in R or Excel, and then pop the results into Datawrapper for an easily understandable chart and send my coworker the URL along with the summary table. When they get that quick, first result, they can ask me to dive deeper, to go in a different direction, or stop there having enough of an answer for what they needed.
Sometimes, the result might be meaningful enough that we'll want to further develop it into a graphic for social media, a blog post, or a report. There are lots of options for where things can go, but the speedy Datawrapper chart is a very important first step.
What's your guiding principle when working on data visualizations?
Understand the context for the question! Why is it being asked, why is it important? What is the question behind the question?
Is what I'm working on exploratory or explanatory? If it's a quick(ish) answer to an internal question, it's often more exploratory. My teammate might want a fuller picture and to look at a couple dimensions of what they're asking. But if it's moved to the stage of publishing a data viz externally, we're in the explanatory realm. We need to think about simplifying, clarifying, annotations and takeaways. How will we either use the title to make our point clear, or situate the chart/map in a context that makes it clear? I believe that in most cases, our audience is going to spend just a few seconds looking at a data visualization before moving on, unless it makes something click very quickly for them. So we need to get our point across very quickly and clearly.
One thing I love about Datawrapper is that I can use tooltips to provide additional data points for the users who want to dig in more than that initial glance. I also use the notes to add any critical methodology information for the minority of users who want that level of detail about how we did our analysis, while deprioritizing it in the visual hierarchy so that most users can focus on the story.
What's your favorite Datawrapper feature?
Tooltips. It's what makes Datawrapper so much more valuable than the kinds of static charts and maps that I can pull together pretty easily. Datawrapper will output a clean and beautiful chart or map but offer a level of interactivity that provides so much additional helpful information without cluttering things up or requiring hours and hours of coding beyond my technical abilities.
We're looking forward to Lucy's talk at Unwrapped! Until then, you can find more of her work on her ANHD page. To sign up for Unwrapped and hear Lucy and other great speakers, visit our conference website.
Comments